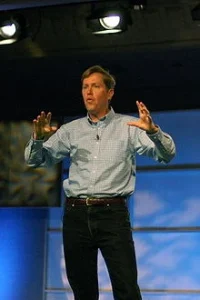
Jeff Hawkins
Jeff Hawkins is a visionary inventor, neuroscientist, and entrepreneur who has made profound contributions to the fields of neuroscience and technology. Born in 1957, he is best known for his groundbreaking work in understanding the brain's architecture and applying those insights to create innovative technologies. Hawkins co-founded Palm Computing and Handspring, pioneering the development of handheld computing devices like the Palm Pilot and Treo smartphones. However, his true passion lies in neuroscience. He developed the influential Memory-Prediction Framework, proposing that the brain operates by making predictions about the world. In 2002, Hawkins founded the Redwood Center for Theoretical Neuroscience, where he continues to explore the brain's mysteries. His 2004 book, "On Intelligence," further elucidated his theories on the brain and its implications for artificial intelligence. Jeff Hawkins remains a visionary thinker at the intersection of neuroscience and technology, leaving an indelible mark on both fields.
Books Mentioned on the Lex Fridman Podcast #25 - Jeff Hawkins
Exploring the Human Brain and AI: Insights from Jeff Hawkins on the Lex Fridman Podcast
Jeff Hawkins, a renowned figure in the field of neuroscience and artificial intelligence, has made significant contributions to understanding the human brain and its potential emulation in AI. He founded the Redwood Center for Theoretical Neuroscience in 2002 and Numenta in 2005. His notable work includes the 2004 book “On Intelligence” and research focusing on reverse-engineering the neocortex. Hawkins’ team has developed concepts like Hierarchical Temporal Memory (HTM) and the Thousand Brains Theory of Intelligence.
Hierarchical Temporal Memory (HTM) and Its Evolution
Hierarchical Temporal Memory, introduced in 2004, represents a pioneering approach in AI, inspired by the structure and function of the human neocortex. HTM’s design mirrors the hierarchical and temporal aspects of neural processing in the brain, aiming to create more human-like intelligence in machines. This concept has evolved over time, reflecting advancements in understanding the neocortex and its complex mechanisms.
Thousand Brains Theory of Intelligence
The Thousand Brains Theory, a more recent development in Hawkins’ work, proposes a revolutionary model for understanding intelligence. It suggests that intelligence arises from the interaction and integration of numerous ‘mini-brains’ or modules within the neocortex. This theory challenges traditional views of a centralized intelligence system, offering a new perspective on how the brain processes and interprets information.
Impact on Artificial Intelligence
Hawkins’ theories have significantly influenced the AI community, offering new directions for developing intelligent systems. By drawing parallels between the human brain’s functioning and potential AI architectures, these theories provide a roadmap for creating more advanced, efficient, and human-like AI.
The Complexity of Neural Processes and AI Limitations
In the second segment of his conversation with Lex Fridman, Jeff Hawkins delves deeper into the intricacies of neural processes, emphasizing how real neurons differ significantly from artificial ones. He explains that real neurons are predictive engines capable of recognizing dozens to hundreds of unique patterns, a complexity not mirrored in current AI models. This difference underscores the limitations of current AI systems, particularly in areas like learning efficiency and robustness against adversarial attacks.
Sparse Representations in AI and the Thousand Brains Theory
Hawkins highlights the potential of introducing sparseness into artificial neural networks, inspired by how the brain operates. Sparse representations, where only a small percentage of neurons are active at any given time, contribute to the robustness and efficiency of the brain’s processes. He also touches upon the Thousand Brains Theory, suggesting a model where numerous ‘mini-brains’ within the neocortex work in concert. This concept could revolutionize AI’s approach to learning and problem-solving.
The Interplay of Learning and Inference in AI and the Human Brain
An intriguing part of the conversation revolves around the simultaneous nature of learning and inference in the human brain, as opposed to the distinct stages in artificial neural networks. Hawkins stresses the importance of continuous learning in AI, a feature inherent to the brain’s functionality. He also discusses the potential for AI to scale beyond human intelligence in specific domains, though he cautions against directly equating this with a comprehensive understanding or emulation of human intelligence.
Challenges and Ethical Considerations in AI Development
As the discussion progresses, Hawkins acknowledges the challenges and ethical considerations in AI development. He emphasizes the importance of focusing AI research on replicating the neocortex’s intelligence aspects, avoiding human-like emotional or reproductive traits. The conversation also touches on existential risks associated with AI, with Hawkins expressing skepticism about the likelihood of such threats materializing, provided AI development remains focused and responsible.
The Essence of Neural Function and AI Applications
In the final part of his discussion with Lex Fridman, Jeff Hawkins delves into the essence of neural functions, contrasting them with artificial neural networks. He emphasizes the complexity of real neurons as predictive engines, capable of recognizing numerous patterns, a capability that current AI models lack. Hawkins discusses the significance of sparse representations in AI, inspired by the brain’s efficiency in utilizing a small percentage of neurons at any given time. This approach could lead to more robust and efficient AI systems.
Continuous Learning and Multimodal AI
Hawkins highlights the importance of continuous learning in AI, drawing parallels with the human brain’s simultaneous nature of learning and inference. This approach differs from the distinct stages seen in artificial neural networks and could lead to AI systems that learn more efficiently and adaptively. He also touches on the potential for AI to handle multimodal inputs, a feature inherent to human intelligence but largely unexplored in current AI models.
Ethical Considerations and Future Directions in AI
The conversation also covers the ethical considerations and challenges in AI development. Hawkins suggests focusing AI research on replicating the intelligence aspects of the neocortex, avoiding human-like emotional traits. He expresses skepticism about existential risks associated with AI, provided its development remains focused and responsible.
The Role of AI in Understanding the Universe
Hawkins envisions AI playing a crucial role in understanding the universe and addressing the fundamental questions of existence. He believes that creating intelligent machines, not necessarily human-like, could be the key to unlocking mysteries that are currently beyond human comprehension.
Conclusion
Jeff Hawkins’ insights provide a profound understanding of the potential and limitations of AI. His views on continuous learning, sparse representations, and ethical considerations in AI research offer valuable guidance for future advancements in the field. His optimism about AI’s role in understanding the universe and addressing existential questions highlights the transformative impact of AI beyond mere technological advancements.