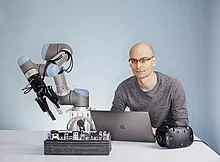
Pieter Abbeel
Pieter Abbeel is s a professor of electrical engineering and computer sciences, Director of the Berkeley Robot Learning Lab, and co-director of the Berkeley AI Research (BAIR) Lab at the University of California, Berkeley. He is also the co-founder of covariant.ai, a venture-funded start-up that aims to teach robots new, complex skills, and co-founder of Gradescope, an online grading system that has been implemented in over 500 universities nationwide. He is best known for his cutting-edge research in robotics and machine learning, particularly in deep reinforcement learning.
Books Mentioned on the Lex Fridman Podcast #10 - Pieter Abbeel
Exploring Deep Reinforcement Learning with Pieter Abbeel and Lex Fridman
In a fascinating episode of the Lex Fridman Podcast, Pieter Abbeel, a renowned professor at UC Berkeley and the director of the Berkeley Robotics Learning Lab, delves into the intricacies of deep reinforcement learning and its applications in robotics. This episode, part of an MIT course on artificial general intelligence, offers profound insights into the future of AI and robotics.
The Intersection of Hardware and Software in AI
Abbeel begins by addressing a question about when robots might be able to beat a top tennis player like Roger Federer. He highlights the dual challenges in AI: while software advancements are crucial, hardware limitations currently impede the physical capabilities of robots. The discussion extends to the feasibility of robots performing complex physical tasks, like playing tennis, where both hardware and software intricacies play significant roles.
The Progress and Challenges of Deep Reinforcement Learning
The conversation shifts to the realm of deep reinforcement learning (RL). Abbeel explains the complexities involved in reinforcement learning, particularly when rewards for actions are sparse or delayed. He sheds light on the need for vast experiences to discern patterns leading to higher rewards, highlighting the efficiency of policy gradient updates in learning from these experiences.
The Role of Neural Networks in Reinforcement Learning
Delving deeper, Abbeel discusses the role of neural networks in reinforcement learning. He describes them as gradual tilers of space, benefiting from linear control aspects and sharing expertise across various controllers. This approach, he suggests, has been pivotal in the success of deep RL, though he acknowledges the challenges it faces in scaling to more complex, real-world problems.
Hierarchical Reasoning in AI
The conversation then explores hierarchical reasoning in AI. Abbeel notes that while hierarchical systems have been conceptualized in AI for decades, their integration with real-world perception and action is still in its infancy. He discusses different approaches to imbue AI with hierarchical reasoning, from bolting deep learning onto traditional models to exploring information-theoretic approaches and meta-learning.
The Promise and Limitations of Transfer Learning
As the dialogue progresses, Abbeel reflects on the concept of transfer learning. He acknowledges its significant advancements, particularly in deep learning, where models trained on large datasets can be fine-tuned for specific tasks. However, he also points out the nuanced levels of generalization and the challenges in achieving a form of generalization that goes beyond pattern recognition to encompass fundamental understanding, akin to physics.
Reinforcement Learning’s Role in Autonomous Vehicles and Robotics
Finally, the discussion turns to the application of reinforcement learning in autonomous vehicles and robotics. Abbeel debates the merits of imitation learning versus self-play, emphasizing the latter’s potential in providing more consistent learning signals. He also touches upon the role of simulation in AI training, suggesting that an ensemble of simulators could provide a more comprehensive learning environment than a single, highly accurate simulator.
Delving Deeper into AI and Robotics with Pieter Abbeel and Lex Fridman
In this segment of the Lex Fridman Podcast, Pieter Abbeel continues his fascinating discussion, touching upon the possibility of robots participating in sports like tennis. He emphasizes the current limitations in robot hardware and the significant role of software in overcoming these challenges. This leads to an intriguing exploration of whether robots could realistically perform complex physical activities such as playing tennis, considering both hardware and software intricacies.
Impressions and Challenges in Robotics
Abbeel shares his admiration for the advancements in robotics, particularly highlighting Boston Dynamics’ achievements. The conversation then shifts to the psychological aspects of interacting with robots, discussing how humans tend to anthropomorphize robots, attributing human-like qualities to them. This segment brings to light the unique challenges in the field of robotics, from the physical capabilities of robots to their interaction with humans.
Reinforcement Learning and Human Interaction
The discussion delves into the potential of reinforcement learning (RL) to incorporate human-like interactions. Abbeel explores the idea of RL systems optimizing their objectives to be more interactive and personable, effectively learning to be more engaging and likable to humans. This concept opens up possibilities for AI to not only perform tasks but also to interact in a more human-centric manner.
Transfer Learning and Generalization in AI
A significant portion of the conversation is dedicated to the concept of transfer learning. Abbeel acknowledges the advancements in this area, especially in deep learning, where large-scale models can be fine-tuned for specific tasks. However, he also highlights the challenges in achieving true generalization that extends beyond pattern recognition to a fundamental understanding of underlying principles, similar to how physics explains natural phenomena.
Applications in Autonomous Vehicles and Robotics
The role of reinforcement learning in autonomous vehicles and robotics garners attention. Abbeel discusses the merits of different learning approaches, such as imitation learning versus self-play, and their potential impact on autonomous systems. The conversation also touches upon the role of simulation in AI training, suggesting that a combination of multiple simulators could provide a more comprehensive learning environment.
The Future of AI Safety and Ethical Considerations
Towards the end of this segment, the conversation shifts to the crucial topic of AI safety and ethics. Abbeel discusses the importance of ensuring safety in AI and robotics, particularly as these technologies increasingly interact with the physical world. He ponders on the methodologies and strategies required to systematically approach AI safety, drawing parallels with human processes such as driving tests.
Conclusion
This part of the podcast offers deep insights into the challenges and potentials of AI and robotics. From exploring the feasibility of robots in physical tasks to discussing the ethical and safety aspects of AI, Pieter Abbeel and Lex Fridman navigate through a myriad of topics, presenting a thought-provoking discourse on the future of AI.